Quantum AI Olympics
A research team from Perimeter, Zapata AI, and Vector Institute sets up a “race” between quantum and classical AI models.
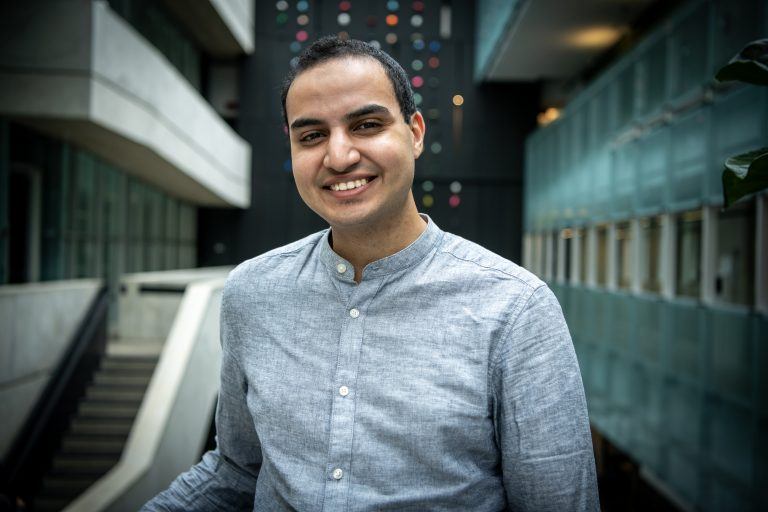
Imagine going to Olympic hurdle races, where, instead of human runners, the competitors are different kinds of artificial intelligence (AI) models – some run on regular or “classical” computers and others on quantum computers.
Depending on the task or the size of the hurdle, the quantum model is sometimes the winner. Other times, the classical computer AI is the winner.
This is exactly the competitive framework that a team of researchers, including Perimeter Scholars International (PSI) Fellow Mohamed Hibat-Allah, set up in a paper published this week in Communications Physics.
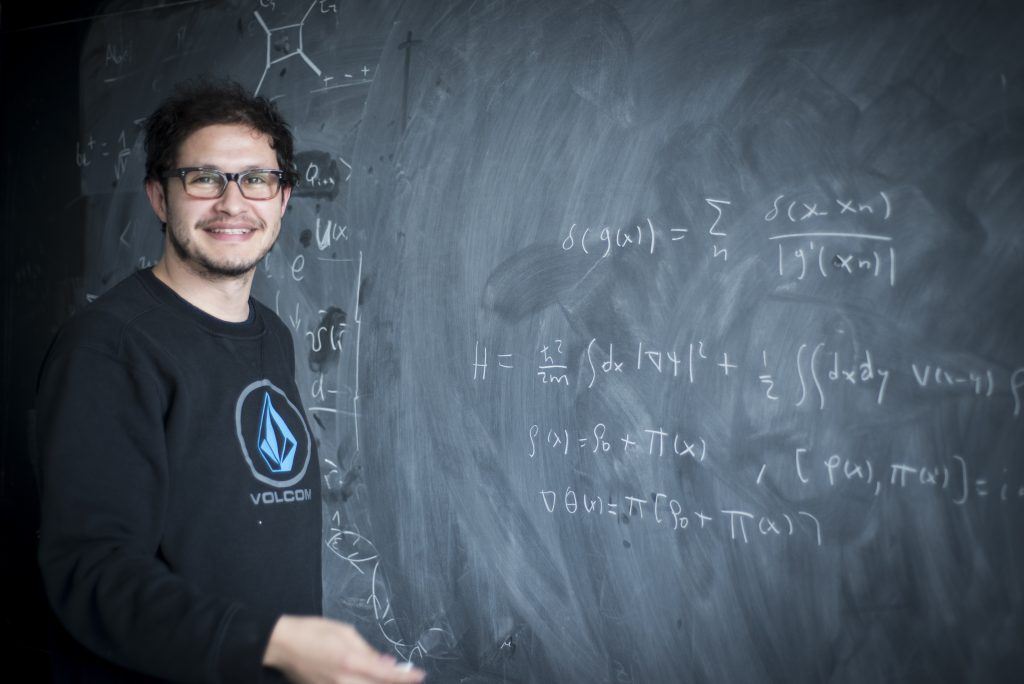
The paper was co-authored with Juan Carrasquilla, a former Perimeter postdoc and current Visiting Fellow, who became a faculty member at the Vector Institute for Artificial Intelligence in Toronto and is now at ETH Zurich. Marta Mauri and Alejandro Perdomo-Ortiz of Zapata AI, an industrial generative AI company, were also co-authors.
Quantum generative AI, which is at the crossroads of quantum computing and generative artificial intelligence (similar to those at the heart of applications like ChatGPT), is garnering intense interest in both industry and academia. The hope is to use quantum computers to speed up or improve upon generative AI models – and vice versa, to use generative AI to make quantum computer algorithms more efficient.
The recent paper by Hibat-Allah and his colleagues is based on previous work done by members of the Zapata AI team that has been developing metrics to measure “generalization,” the ability of an AI system to extrapolate and go beyond the data that is supplied in the training.
Generalization is the goal for anyone using a quantum system or AI system for a practical application. Whether one is trying to solve an optimization problem, for example, or simulate the chemistry for a new drug, the hope is to get the system to extrapolate high-quality and groundbreaking new insights from the training set.
In this paper, the researchers were able to demonstrate for the first time that quantum generative models could outperform classical AI in the context of data generalization. Specifically, they showed that quantum generative models can win in regimes where training data is scarce.
The practical quantum advantage (PQA) race: a sports analogy.
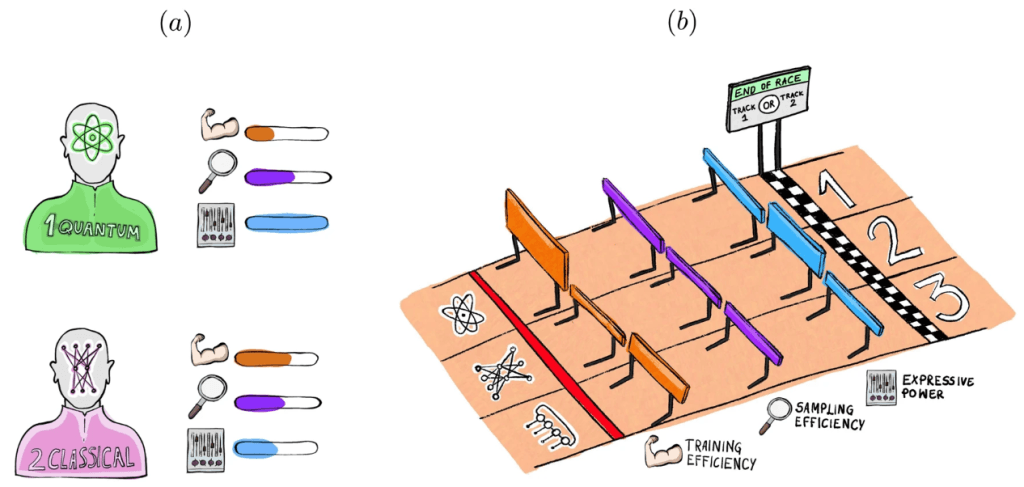
“We found that in the scarce data regime, the quantum models provide the best generalization power when compared to the classical models,” Hibat-Allah says.
This is useful in the pharmaceutical industry, for example, where the datasets for new molecules that the AI can be trained on would be thin.
“This is highly desirable in certain types of real-world applications,” Hibat-Allah says. “One prominent example is drug discovery. Being able to generate new and high-quality drugs by extrapolating from a small training dataset of drug molecules can be a game-changer.”
Hibat-Allah makes it clear that the research team was simulating a quantum computer using a classical computer, rather than an actual quantum computer.
In a quantum computer, subatomic particle states (such as spin) are in a quantum mechanical state known as superposition. This strange feature of quantum mechanics generates quantum bits or “qubits,” that can potentially enable quantum computers to gain an advantage in solving certain types of problems much more efficiently than regular computers.
The problem is that quantum computations are difficult to control because these superpositions are extremely fragile and will collapse if there is any “noise” from the outside or from the interactions of many qubits.
But Hibat-Allah says it is meanwhile possible for researchers to take advantage of what are known as “tensor networks,” which can be used to simulate quantum circuits. “There is a relationship between these tensor networks and quantum circuits,” he says.
Essentially, they created a framework – a series of races – pitting the best of quantum AI against the best of classical AI models. The goal was to identify the best way to achieve practical quantum advantage in different situations.
There is more work to be done. Hibat-Allah says the hope is to raise the bar to include the expected noise in a quantum system and increase the number of qubits that are being simulated, in order to get even more accurate metrics.
“A promising future research direction is to investigate the impact of noise on our results and to implement our method on real quantum devices with a larger number of qubits,” Hibat-Allah says.
It is also possible to set up even more exciting competitions, where quantum and classical models are helping each other out in relay-type races.
“We can train those architectures to the limits of what can be achieved by classical computers and then pass the torch to quantum computers when they are ready in the future,” says Hibat-Allah. This idea is outlined in another Nature Communications paper by the Zapata AI researchers in December.
As a PSI Fellow at Perimeter, Hibat-Allah is able to combine research, teaching, and administrative work.
The work on this paper is part of his research, but he is also doing work using language models to simulate quantum systems. “You can imagine a quantum system that has many degrees of freedom as being like the words of a sentence in a language model,” he says. “In my other research, we are trying to push this analogy to improve our understanding of quantum systems.”
Another part of being a PSI Fellow involves teaching the PSI master’s students. “I am very grateful for this opportunity. It reminds me of what it is like to be a student,” he says.
The administrative part of his work includes being involved in various committees such as the career trajectories working group at Perimeter. “I find that very rewarding,” he adds.
Hibat-Allah is originally from Morocco, but he completed his undergraduate degree in France at the Ecole Normale Superieure de Lyon prior to coming to Perimeter to finish a master’s degree through PSI. He then completed his PhD between the University of Waterloo and Vector Institute, with Carrasquilla as his supervisor and Perimeter Associate Faculty member Roger Melko as his co-supervisor. He became a PSI Fellow at Perimeter last August.
Hibat-Allah is in an invigorating field at an incredible juncture in history when both AI and quantum computers are progressing and evolving at a rapid pace.
“If we can manage to use real quantum devices to demonstrate a practical advantage compared to powerful classical models, that would be really exciting for me,” he says.